Human Motion Prediction Metrics: from Time to Frequency

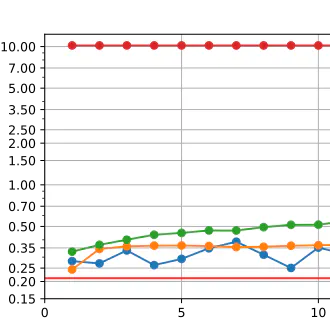
Collaborative robotics has the potential to revolutionize industrial applications by integrating human and robot capabilities. However, for efficient and seamless collaboration, predicting human motion is essential. This allows robots to dynamically adjust their behavior and avoid potential collisions. Despite significant progress in this field in recent years, there is still uncertainty surrounding the metrics needed for a complete and accurate evaluation of algorithm performance. Currently, the evaluation of Human Motion Prediction (HMP) techniques is based on metrics focusing exclusively on geometric aspects. This work proposes a HMP metric to evaluate the realism and naturalness of predicted human motion sequences based on their frequency spectra. Using the Human 3.6M dataset, several experiments were conducted to demonstrate the effectiveness of the proposed metric. The results showed the ability of this metric to capture insights related to the realism of the predicted motion sequences, making it a valuable complementary tool alongside existing metrics for evaluating HMP algorithms.
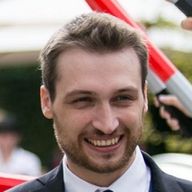