Towards Explainable Human Motion Prediction in Collaborative Robotics

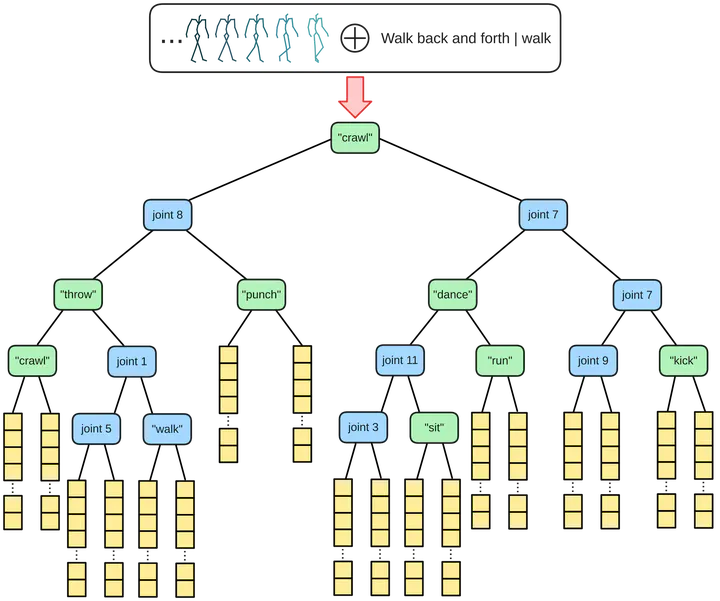
Predicting human motion is challenging due to its complex and non-deterministic nature. This is particularly true in the context of Collaborative Robotics, where the presence of the robot significantly influences human movements. Current Deep Learning models excel at modeling this complexity but are often regarded as black boxes. Explainable Artificial Intelligence (XAI) offers a way to interpret these models. In this work, we introduce an XAI approach to identify key features in a Human Motion Prediction (HMP) system. Additionally, we semantically associate action labels to the joint rotations representing human motion to further improve the interpretability and precision of the model. We evaluated our system using the AMASS dataset and BABEL labels. Experimental results demonstrated the importance of specific action-related features, enhancing prediction accuracy compared to the Zero-Velocity baseline model.